Booming Artificial Intelligence Techniques
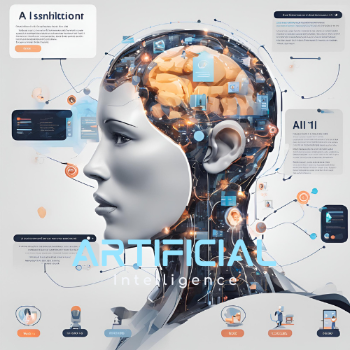
Lets explore the fascinating and rapidly evolving world of artificial intelligence (AI). AI is a field with the capability to perform tasks typically requiring human intelligence, such as decision-making, speech recognition, and visual perception. From robotics and information technology (IT) to research and development, AI is revolutionizing numerous industries. In this blog, we’ll delve into the latest techniques and advancements in AI, providing a comprehensive overview of the current state of the field and its future directions.
AI Techniques and Their Evolution
Generative AI
Definition:
- Generative AI focuses on creating new data samples that are similar to existing data, using models that can generate realistic data points.
Generative Models
- Generative Adversarial Networks (GANs):
Introduced by Ian Goodfellow in 2014, GANs consist of two neural networks, a generator and a discriminator, trained simultaneously. The generator creates data, and the discriminator evaluates it against real data, aiming for the generator to produce data indistinguishable from real data.
- Variational Autoencoders (VAEs):
These models encode input data into a latent space and then decode it back to the original data space. They introduce a probabilistic approach to model the data generation process, allowing for the creation of new, similar data points.
- Autoregressive Models:
Examples include PixelRNN and PixelCNN, which generate data one step at a time, with each step conditioned on the previous ones. This method has been particularly successful in generating images and sequences.
Applications of Generative AI
- Image Generation:
GANs have been used to create highly realistic images. Projects like StyleGAN have demonstrated the ability to generate high-fidelity images of faces, landscapes, and more.
- Text Generation:
Models like GPT (Generative Pre-trained Transformer) by OpenAI have revolutionised text generation, producing coherent and contextually relevant paragraphs of text. These models have applications in chatbots, content creation, and more.
- Music and Audio:
Generative models can create music compositions, synthesise human-like voices, and generate sound effects. Projects like OpenAI’s MuseNet and Jukedeck have shown promising results in this area.
Evolution of Generative AI
- Early Methods:
– Initial generative models were relatively simple, such as Gaussian Mixture Models (GMMs) and Hidden Markov Models (HMMs), which were limited in the complexity of the data they could generate.
– Autoencoders, which compress data into a latent space and then reconstruct it, laid the groundwork for more sophisticated models.
- Breakthrough with GANs and VAEs:
– GANs and VAEs represented significant advancements in generative modelling. GANs, in particular, brought a new paradigm of adversarial training, which significantly improved the quality of generated data.
– VAEs introduced a probabilistic approach to generation, allowing for more controlled and diverse outputs.
- Deep Learning and Transformers:
– The advent of deep learning and convolutional neural networks (CNNs) enhanced the capabilities of generative models, particularly in image generation.
– The development of transformer architectures, such as those used in models like GPT-3, enabled significant advancements in text generation, allowing for longer, more coherent outputs.
- Recent Advancements:
– Advances in computing power and the availability of large datasets have driven progress in generative AI.
– Techniques like StyleGAN have refined the ability to generate high-resolution, realistic images.
– Improvements in training stability and efficiency have made it possible to train larger and more complex generative models.
Challenges and Ethical Considerations
- Quality and Realism: While generative models have made impressive strides, ensuring consistently high quality and realism in generated data remains a challenge.
- Control and Diversity: Balancing control over the generation process with the diversity of outputs is crucial. Models should generate varied data while allowing users to guide the generation process.
- Ethical Concerns:
- – Misuse: Generative AI can be used to create deep fakes, fake news, and other malicious content.
- – Bias: Generated data can perpetuate biases present in the training data, leading to ethical concerns in applications like hiring or law enforcement.
- – Intellectual Property: The use of AI-generated content raises questions about ownership and copyright.
Future Directions of AI
- Improved Models:Continued research aims to develop more robust and efficient generative models, capable of producing even higher quality and more diverse outputs.
- Integration with Other AI: Combining generative models with other AI techniques, such as reinforcement learning, could open up new possibilities for applications.
- Ethical Frameworks: Developing frameworks to address ethical issues, including transparency, accountability, and fairness, is crucial as generative AI becomes more prevalent.
- Practical Applications: Expanding the practical applications of generative AI in fields like medicine (e.g., drug discovery), finance (e.g., algorithmic trading), and personalised content creation will likely drive future advancements.
Booming AI Techniques: Latest Updates
- Reinforcement Learning (RL)
Definition:
Reinforcement learning is a type of machine learning where an agent learns to make decisions by performing actions and receiving feedback from the environment in the form of rewards or penalties.
Advancements:
– Deep Reinforcement Learning:Combining deep learning with RL has led to breakthroughs in areas such as game playing (e.g., AlphaGo) and robotics.
– Applications:RL is used in autonomous vehicles, robotic control, resource management, and complex decision-making tasks.
- Explainable AI (XAI)
Definition:
Explainable AI focuses on creating AI models whose decisions can be understood and interpreted by humans.
Advancements:
– Interpretable Models: Developing models that are inherently interpretable or using techniques to explain black-box models.
– Applications: XAI is crucial in domains like healthcare, finance, and law, where understanding the rationale behind AI decisions is essential.
- Federated Learning
Definition:
Federated learning is a distributed machine learning approach that enables training models across multiple decentralised devices or servers while keeping the data localised.
Advancements:
– Privacy-Preserving AI: By keeping data on local devices, federated learning enhances privacy and security.
– Applications: Used in industries like healthcare, finance, and IoT, where data privacy is paramount.
Conclusion:
Artificial Intelligence is an ever-evolving field with continuous advancements transforming various industries. As AI technology continues to grow, addressing challenges related to quality, control, and ethics will be crucial. The future of AI looks promising, with ongoing research and innovation paying the way for more sophisticated & ethical.