Edge Artificial Intelligence
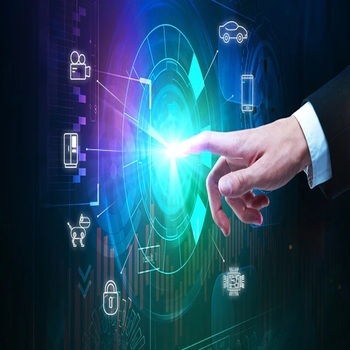
As per the Gartner’s Report, Edge AI will be one of the technologies dominating the future. Let’s learn something about how Edge AI can be viewed from a research perspective.
Edge Artificial Intelligence (AI)
Edge AI is the deployment of AI applications in devices throughout the physical world. It’s called “edge AI” because the AI computation is done near the user at the edge of the network, close to where the data is located, rather than centrally in a cloud computing facility or private data center. Edge AI speeds up decision-making, makes data processing more secure, improves user experience with hyper-personalization, and lowers costs — by speeding up processes and making devices more energy efficient. Edge AI focuses on best practices, architectures and processes for extending data science, machine learning and AI outside the cloud.
Research perspective of Edge AI
In research perspective, Edge AI can be used in the following use cases:
- Powering smart hospitals
- Autonomous Vehicles
- Industrial Internet of Things (IIoT) and
- Drones
Edge AI can combine the power of the cloud with the benefits of local operation to improve the performance of AI algorithms over time.
Powering smart hospitals
Edge AI applications help with improved security of data which is a necessity for smart hospitals to function effectively. AI Healthcare applications such as remote surgery and diagnostics, as well as monitoring of patient vital signs are mostly based on edge devices that perform AI at the edge. Doctors can use a remote platform to operate surgical tools from a distance where they feel safe and comfortable. Companies are able to use Edge AI to perform medical tasks like high precision thermal screening, inventory management, remote monitoring of patients, and even prediction of ailments.
Autonomous Vehicles
Autonomous vehicles (or self-driving vehicles) are a prime example of edge AI applications in the modern world. They make use of Edge AI-enabled devices that allow the processing of data on the same hardware. This processing ability within the same hardware is extremely important as the swift detection and identification of objects is required to prevent fatal accidents. In an autonomous navigation system the AI algorithms are trained in the cloud, but the inference is run on the car for controlling the steering, acceleration and brakes. Data scientists develop better self-driving algorithms in the cloud and push these models to the vehicle. In some cars, these systems continue to simulate controlling the car even when a person is driving. The system then notes when the human does something the AI did not expect, captures relevant video and uploads this to the cloud to improve the algorithm. The main control algorithm is then enhanced using input from all the vehicles in the fleet, which is pushed out in the next update.
Industrial Internet of Things (IIoT)
With Edge AI, Industrial Internet of Things devices can carry out visual inspections and facilitate the faster control of robotic activities and all these at lower costs.
The above Edge AI applications show that lives, work, and businesses all stand to gain improvement when edge computing is combined with artificial intelligence for improving customer experiences, eliminating risk to humans, augmenting human efforts in healthcare, and making roads safer.
Drones
Edge AI applications in drones can be used for several things like traffic monitoring, construction, and cartography. Drones make use of image recognition, visual search, object detection, and tracking. Artificial intelligence-powered drones carry out object detection and identification activities by mimicking actual human visual search behavior.
Benefits of Edge AI
The benefits of edge AI include:
Intelligence: AI applications are more powerful and flexible than conventional applications that can respond only to inputs that the programmer had anticipated.
Real-time insights: Since edge technology analyses data locally rather than in a faraway cloud delayed by long-distance communications, it responds to users’ needs in real time.
Reduced cost: By bringing processing power closer to the edge, applications need less internet bandwidth, greatly reducing networking costs.
High availability: Decentralization and offline capabilities make edge AI more robust since internet access is not required for processing data.
Persistent improvement: AI models grow increasingly accurate as they train on more data. When an edge AI application confronts data that it cannot accurately or confidently process, it typically uploads it so that the AI can retrain and learn from it.