Synthetic Data
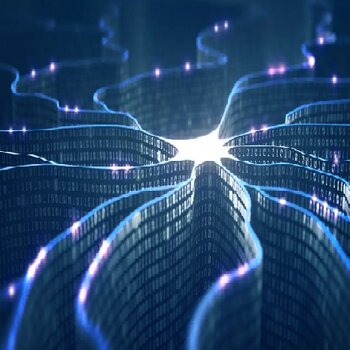
In the ever-expanding world of research, data plays a pivotal role in unlocking insights, validating hypotheses, and driving scientific progress. However, the availability of high-quality and privacy-sensitive data has become a significant challenge. This is where synthetic data emerges as a game-changing solution.
Synthetic data, a concept gaining increasing recognition, refers to artificially generated data that mimics the statistical properties of real data while ensuring privacy and confidentiality. Additionally, with its growing importance, synthetic data offers researchers a valuable tool to overcome limitations related to data scarcity, privacy concerns, and restricted access to sensitive datasets.
In this blog, we will explore the fascinating world of synthetic data and its relevance in research. Furthermore, we will delve into its benefits, limitations, and potential future implications. By adopting innovative approaches like synthetic data, researchers can unlock new frontiers in their respective fields while ensuring ethical and privacy-conscious practices.
Understanding Synthetic Data
Synthetic data replicates real data’s statistical properties while protecting privacy. It is generated using algorithms like GANs or VAEs, it mirrors distributions, correlations, and patterns. This allows researchers to work with sensitive data while safeguarding identities and complying with regulations.
Synthetic Data in Research Perspectives
Synthetic data offers significant benefits for research.
- Overcoming Data Scarcity: Synthetic data addresses limited data availability, expanding sample size and diversity for enhanced statistical power and generalizability.
- Privacy Preservation: It generates new data unlinked to individuals, ensuring confidentiality and enabling research on sensitive topics.
- Exploration of Sensitive Scenarios: Synthetic data enables the study of rare or sensitive events, facilitating research on difficult or unethical observations.
- Unlocking New Possibilities for Analysis and Modeling: It provides a controlled environment for hypothesis testing, model development, and methodological advancements across research domains. Consequently, researchers can leverage synthetic data’s potential to make significant strides in their research and contribute to scientific discovery.
Advantages of Synthetic Data in Research
- Controlled Testing and Simulations: Synthetic data allows researchers to conduct controlled experiments and simulations by generating datasets with known properties. As a result, they can rigorously test and validate findings before costly real-world trials, such as simulating clinical trials in healthcare research.
- Reproducibility and Scalability: Synthetic data ensures reproducibility and scalability by providing standardized data representations. By sharing these representations, researchers enable others to reproduce experiments and validate findings. Moreover, synthetic data enables the generation of large datasets, particularly useful in fields with limited real-world data, ensuring robust findings.
- Data Sharing and Collaboration: Synthetic data facilitates data sharing and collaboration while addressing privacy concerns. Researchers can exchange synthetic datasets without compromising sensitive information, fostering collaboration across institutions, disciplines, and geographies. This Interdisciplinary research benefits from synthetic data, as it provides a shared dataset with statistical properties relevant to each discipline and leads to innovative insights and solutions.
- Insights from a Recent Podcast: Synthetic data has the potential to address privacy concerns and serve as a comprehensive solution for AI data needs, as discussed by Alexy Thomas from EY India in a recent podcast. It overcomes limitations of actual data, enhances AI training and testing, enables faster iterations and deployment of AI systems, and has applications in evaluating market behavior and developing innovative products, benefiting the financial services sector.
Therefore, synthetic data offers significant advantages in research. It provides a controlled testing environment, enhances reproducibility and scalability, and enables data sharing and collaboration. Leveraging these advantages unlocks new possibilities and drives meaningful progress in various fields.
The Future of Synthetic Data in Research Perspectives
The future of synthetic data in research is promising and impactful. Advancements in generative models allow for the creation of synthetic data that closely resembles real data. Here’s what you need to know:
- Accelerating Innovation: Synthetic data enables researchers to experiment and iterate quickly, leading to faster advancements and discoveries.
- Overcoming Data Scarcity and Privacy Concerns: Synthetic data offers an alternative when access to real-world datasets is limited due to privacy regulations or data availability.
- Mitigating Bias and Ethical Considerations: Synthetic data helps create balanced and unbiased datasets, reducing bias and ensuring fair and ethical research practices.
- Enabling Generalization and Transfer Learning: Synthetic data aids in developing robust and adaptable AI models that can handle diverse inputs and transfer knowledge across domains.
- Enhancing Robustness and Resilience: Synthetic data allows researchers to evaluate model performance, identify vulnerabilities, and strengthen AI systems for real-world applications.
The potential impact of synthetic data is vast across research fields
- Healthcare: Revolutionizing medical research and drug development, synthetic data preserves privacy and enhances precision medicine.
- Social Sciences: Simulating and analyzing complex social phenomena while protecting confidentiality.
- Environmental Studies: Generating models to predict climate change impacts and inform policy decisions.
Synthetic data encourages interdisciplinary collaboration, driving innovative solutions
- Public Health, Urban Planning, and Policy-making: Breakthroughs in these areas can be achieved through collaboration across healthcare, social sciences, environmental studies, and more.
- Finance and Cybersecurity: Synthetic data aids in risk analysis and algorithm testing, ensuring reliability and robustness.
Advancements in synthetic data generation techniques offer new possibilities:
- Addressing Data Scarcity, Privacy Concerns, Bias, and Ethics: Synthetic data helps overcome these challenges.
- Embracing Interdisciplinary Collaboration: Researchers can unlock new insights, solve challenges, and drive scientific progress. The future of synthetic data holds immense potential for research and scientific discovery.
Ethical Considerations and Limitations
When using synthetic data in research, it is important to handle ethical considerations responsibly. Privacy protection is crucial, but we need strong techniques to anonymize the data and reduce the risk of unintended identification. Additionally, researchers must address biases in the training data to ensure unbiased results. However, synthetic data may not accurately capture the complexity of real-world data. Therefore, validation is necessary to ensure that it effectively represents real-world scenarios. To verify the performance and reliability of synthetic data, rigorous validation against real data is essential. It is crucial to use synthetic data for legitimate purposes, adheres to ethical guidelines, and be transparent about the methods used. Documentation plays a key role in fostering transparency and trust while mitigating risks and addressing limitations.
Key Considerations
To navigate the complexities of synthetic data and maintain ethical integrity, researchers should address ethical considerations, acknowledge limitations, and emphasize responsible use, transparency, and documentation. By doing so, they can leverage the benefits of synthetic data for scientific advancement and innovation.
The Path Ahead
Researchers should collaborate to explore synthetic data and improve its methods to achieve reliable outcomes. Synthetic data has the potential to drive breakthroughs and shape the future of research in various fields. By embracing this approach, we can expand our knowledge and make meaningful advancements. Let’s embark on a collective journey of exploration, collaboration, and innovation with synthetic data to contribute significantly to research and societal progress.
Sources
https://www.techtarget.com/searchcio/definition/synthetic-data#:~:text=Synthetic%20data%20is%20information%20that’s,machine%20learning%20(ML)%20models.
https://www.k2view.com/what-is-synthetic-data-generation
https://research.aimultiple.com/synthetic-data-vs-real-data/
https://www.ey.com/en_in/podcasts/tech-trends/2023/03/episode-3-synthetic-data-artificial-data-real-solutions